How machine learning models can amplify inequities in medical diagnosis and treatment
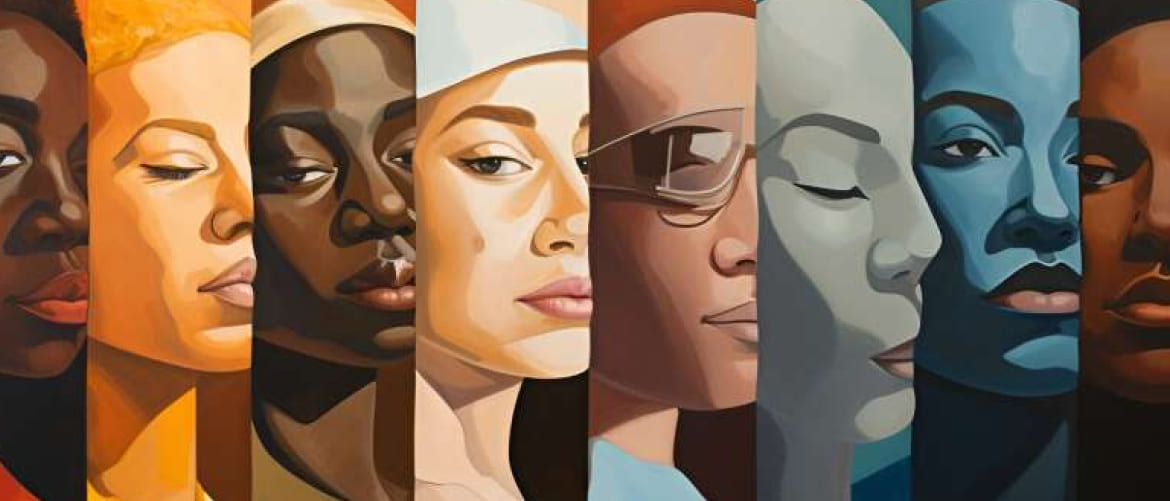
Marzyeh Ghassemi had already begun to wonder whether the use of AI techniques might enhance the biases that already existed in health care long before she commenced her PhD studies at the Massachusetts Institute of Technology. A principal investigator (PI) for AI and health at MIT Jameel Clinic since 2022, she was one of the early researchers to take up this issue, and has been exploring it ever since.
In a new paper written alongside two MIT PhD students, Yuzhe Yang and Haoran Zhang, and fellow MIT Jameel Clinic PI Dina Katabi, Marzyeh has probed the roots of the disparities that can arise in machine learning, often causing models that perform well overall to falter when it comes to subgroups for which relatively few data have been collected and utilised in the training process. In their analysis, the researchers focused on 'subpopulation shifts'—differences in the way machine learning models perform for one subgroup as compared to another. The main point of their inquiry is to determine the kinds of subpopulation shifts that can occur and to uncover the mechanisms behind them so that, ultimately, more equitable models can be developed.